ARJ 2018, 2(1), P.P. 1-12;
archives/2018/Mohammed_Taha/
Review Article
Review of docking and drug-likeness as theoretical and computer aided techniques
Mohammed Taha Yaseen Al-sigar, Ministry of Health, Iraq.*
Introduction
Nearly thirty years ago, the first De Novo method was proposed that could automatically design molecules that optimally complement a protein site based on the three-dimensional structure the receptor [1]. These methods have suffered from two main handicaps,
Poor synthetic feasibility of the suggested molecules,
Lack of predictive power of the scoring functions.
In practice, the molecules selected by De Novo software are generally not the ones that are synthetized. Instead, they are used as inspiration by medicinal chemists to carry out their own designs [2].
The same can be said for docking methods: they owe a large part of their success to user intervention [3]. Under the right conditions, structure-based methods can deliver a 1–20% hit rate, which is ten to several hundred-fold improvement over random high-throughput screening [4]. Similar hit rates have been reported for ligand-based methods [5]. The combination of computational tools and expert users offers a very powerful solution, but it must be acknowledged that the success and ubiquity of virtual screening is due, in large part, to the continuous growth of commercial catalogs, which are easily accessible through public databases such as ZINC [6]. Obtaining one true active out of every 20 molecules tested is perfectly acceptable when the compounds are relatively inexpensive and readily available. But the bar is much higher when truly virtual compounds are explored, because valuable synthetic resources must be invested upfront [7].
*|baghdadpharmacy|@|gmail|.|com
Molecular Docking
Docking is a computational technique that predicts the preferred orientation of one molecule to a second when bound to each other to form a stable complex [8]. Knowledge of the preferred orientation in turn may be used to predict the strength of association or binding affinity between two molecules using scoring functions. In many popular docking methods the ligand is treated as flexible but the protein conformation is kept rigid [9]. Docking plays an important role in the rational design of drugs [10]. During the course of the process, the ligand and the protein adjust their conformation to achieve an overall “best-fit” which is referred to as “induced-fit” [11].
Types of Interactions:
Forces are divided into electrostatic forces, electrodynamics forces, steric forces and solvent-related forces.
Docking approaches:
Two approaches are particularly popular within the molecular docking community. Both approaches have significant advantages as well as some limitations.
Shape complementarity:
An approach uses a matching technique that describes the protein and the ligand as complementary surfaces [12].
Simulation:
This second approach simulates the actual docking process in which the ligand-protein pair wise interaction energies are calculated [13]. In this approach, the protein and the ligand are separated by some physical distance, and the ligand finds its position into the protein’s active site after a certain number of “moves” in its conformational space. The success of a docking program depends on two components: the search algorithm and the scoring function [14].
Mechanics of Docking:
Search algorithm:
The search space in theory consists of all possible orientations and conformations of the protein paired with the ligand. Most docking programs in use account for a flexible ligand, and several attempt to model a flexible protein receptor. Each "snapshot" of the pair is referred to as a pose.
Ligand flexibility:
Conformations of the ligand may be generated in the absence of the76 receptor and subsequently docked7 or conformations may be generated on-the-fly in the presence of the receptor binding cavity [15]. Force field energy evaluations are most often used to select energetically reasonable conformations, but knowledge-based methods have also been used [16].
Receptor flexibility:
Multiple static structures experimentally determined for the same protein in different conformations are often used to emulate receptor flexibility [17].
Scoring function:
The scoring functions are fast approximate mathematical methods used to predict the strength of the non-covalent interaction (also referred to as binding affinity) between two molecules after they have been docked. Most scoring functions are physics-based molecular mechanics force fields (Figure) that estimate the energy of the pose; a low (negative) energy indicates a stable system and thus a likely binding interaction.
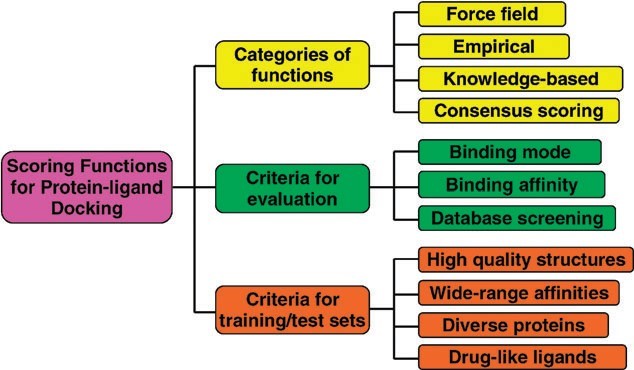
Figure: An illustration of the categories and evaluations of the scoring functions for protein–ligand docking [18].
Applications:
A binding interaction between a small molecule ligand and an enzyme protein may result in activation or inhibition of the enzyme. If the protein is a receptor, ligand binding may result in agonism or antagonism.
Hit identification
– docking combined with a scoring function can be used to quickly screen large databases of potential drugs in silico to identify molecules that are likely to bind to protein target of interest.
Lead optimization
– docking can be used to predict in where and in which relative orientation a ligand binds to a protein (also referred to as the binding mode or pose).
Bioremediation
– Protein ligand docking can also be used to predict pollutants that can be degraded by enzymes [19].
Drug-likeness & bioactivity score
Drug-likeness may be defined as a complex balance of various molecular properties and structural features that determine whether particular molecule is similar to the known drugs. These properties, mainly hydrophobicity, electronic distribution, hydrogen bonding characteristics, molecule size and flexibility and presence of various pharmacophoric features influence the behavior of molecule in a living organism, including bioavailability, transport properties, affinity to proteins, reactivity, toxicity, metabolic stability and many others.
Molinspiration virtual screening toolkit miscreen (version 2011.06)82 is a screening engine for arbitrary target, provided that several active ligands are known. Expert system for calculation of druglikeness score towards GPCR ligands, ion channel modulators, kinase inhibitors, nuclear receptor ligands, protease inhibitors and other enzyme targets based on technology tested on- line.
Drug-likeness Types:
- G protein-coupled receptors (GPCRs): These proteins are active in just about every organ system and present a wide range of opportunities as therapeutic targets in cancer, cardiac dysfunction, diabetes, central nervous system disorders, obesity, inflammation, and pain.
- Ion channels are key components in a wide variety of biological processes that involve rapid changes in cells, such as cardiac, skeletal, and smooth muscle contraction, epithelial transport of nutrients and ions, T-cell activation and pancreatic beta-cell insulin release. In the search for new drugs, ion channels are a frequent target.
- Protein kinases: Phosphorylation is a necessary step in some cancers and inflammatory diseases. Inhibiting the protein kinases, and therefore the phosphorylation, can treat these diseases. Therefore, protein kinase inhibitors are used as drugs.
- Nuclear receptors: Many of these regulated genes are associated with various diseases, which explain why the molecular targets of approximately 13% of U.S. Food and Drug Administration (FDA) approved drugs are nuclear receptors.
- Protease inhibitors (PIs) are a class of antiviral drugs that are widely used to treat HIV/AIDS and hepatitis caused by hepatitis C virus.
- Enzyme inhibitor: blocking an enzyme's activity can kill a pathogen or correct a metabolic imbalance, many drugs are enzyme inhibitors. They are also used as herbicides and pesticides. The most common uses for enzyme inhibitors as drugs are anti-epileptic, male erectile dysfunction, antibiotic and anti-inflammatory drugs [20].
References
1. Danziger DJ, Dean PM. Automated site-directed drug design: a general algorithm for knowledge acquisition about hydrogenbonding regions at protein surfaces. Proc R Soc London Ser B Biol Sci. 1989;236:101–113.
2. Schneider G, Fechner U. Computer-based de novo design of druglike molecules. Nat Rev Drug Discov. 2005;4:649–663.
3. Barril X, Hubbard REE, Morley SDD. Virtual screening in structurebased drug discovery. Mini Rev Med Chem. 2004;4:779–791.
4. Gupta A, Gupta AK, Seshadri K. Structural models in the assessment of protein druggability based on HTS data. J Comput Aided Mol Des. 2009;23:583–592.
5. Horvath D. Pharmacophore-based virtual screening. Methods Mol Biol. 2011;672:261–298.
6. Sterling T, Irwin JJ. ZINC 15–ligand discovery for everyone. J Chem Inf Model. 2015;55:2324–2337.
7. Xavier Barril (2017) Computer-aided drug design: time to play with novel chemical matter, Expert Opinion on Drug Discovery, 12:10, 977-980.
8. Lengauer T; Rarey M: "Computational methods for biomolecular docking". Curr. Opin. Struct. Biol, 1996,. 6 (3): pp.402–6.
9. Yuriev, E; Agostino M. and Ramsland P: Challenges and advances in computational docking: 2009 in review, J. Mol. Recognit, 2011, 24, pp.149–164.
10. Kitchen DB; Decornez H; Furr JR; Bajorath J: "Docking and scoring in virtual screening for drug discovery: methods and applications". Nature reviews. Drug discovery 2004, 3 (11): pp.935–49.
11. Wei BQ; Weaver LH; Ferrari AM; Matthews BW; Shoichet BK: "Testing a flexible-receptor docking algorithm in a model binding site". J. Mol. Biol, 2004 337 (5): pp.1161–82.
12. Meng EC; Shoichet BK; Kuntz ID: "Automated docking with grid-based energy evaluation". Journal of Computational Chemistry, 2004, 13 (4): pp.505–524.
13. Feig M; Onufriev A; Lee MS; Im W; Case DA; Brooks CL: "Performance comparison of generalized born and Poisson methods in the calculation of electrostatic solvation energies for protein structures". Journal of Computational Chemistry, 2004, 25 (2): pp.265–84.
14. Kahraman A; Morris RJ; Laskowski RA; Thornton JM: "Shape variation in protein binding pockets and their ligands". J. Mol. Biol, 2007, 368 (1): pp.283–301.
15. Kearsley SK; Underwood DJ; Sheridan RP; Miller MD: "Flexibases: a way to enhance the use of molecular docking methods". J. Comput. Aided Mol. Des, 1994,. 8 (5): pp.565–82.
16. Friesner RA; Banks JL; Murphy RB; Halgren TA; Klicic JJ; Mainz DT; Repasky MP; Knoll EH; Shelley M; Perry JK; Shaw DE; Francis P; Shenkin PS: "Glide: a new approach for rapid, accurate docking and scoring. 1. Method and assessment of docking accuracy". J. Med. Chem, 2004 47 (7): pp.1739–49.
17. Soldos Z; Reid D; Simon A; Sadjad SB; Johnson AP: "eHiTS: A new fast, exhaustive flexible ligand docking system". Journal of Molecular Graphics and Modelling, 2007, 26 (1): pp.198–212.
18. Hartmann C; Antes I; Lengauer T: "Docking and scoring with alternative side-chain conformations". Proteins, 2009, 74 (3): pp.712–26.
19. http://www.molinspiration.com/
20. Sabah Jawad Salih; Design, Synthesis and Preliminary Pharmacological Evaluation of New 2-Azetidinone Derivatives of Naproxen, Thesis of Ph.D, Univ. of Baghdad, Collage of pharmacy, pp. 26-27.
© 2018 by the authors; licensee ARJ